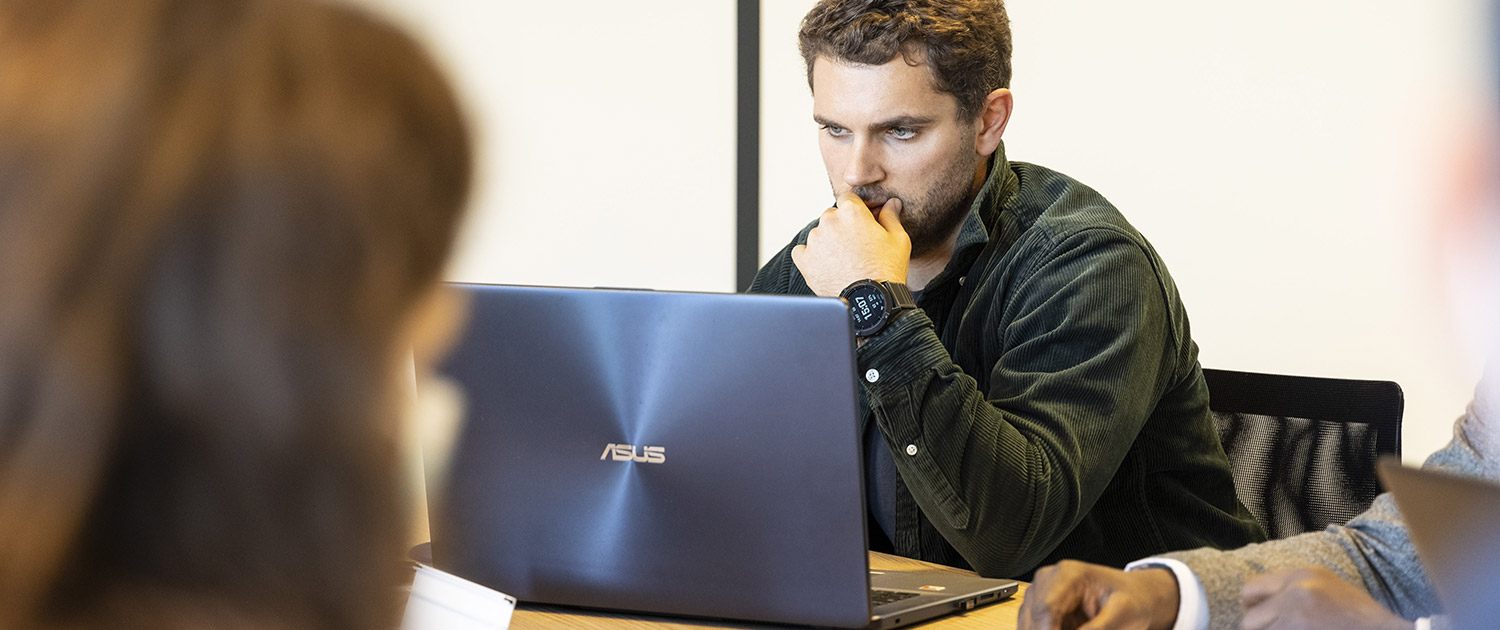
“It is by joining forces for two years that we were able to bring this technology to life—simple, robust, and intelligent.”
AI module and control system: for internal use at SEMOFI
Reinventing geotechnics with machine learning
Geotechnics, a discipline at the core of ground engineering, is undergoing a profound transformation.
As demands for reliability, responsiveness, and sustainability continue to rise, digital tools—particularly machine learning—are becoming powerful drivers of optimization.
The integration of artificial intelligence into soil studies opens up new possibilities: deeper insights into ground conditions, more accurate risk forecasting, and enhanced ability to adapt interventions in real time. In a field traditionally grounded in field expertise and empirical testing, these advancements represent a major turning point.
Towards smarter geotechnical engineering
Machine learning enables a complete rethinking of each phase of the geotechnical study process—making it smoother, more efficient, and more relevant.
Thanks to algorithms’ ability to learn from large datasets, it’s now possible to anticipate ground conditions before even setting foot on site.
By leveraging historical data—whether from past projects or expanded databases—machine learning can:
- Design more targeted testing programs,
- Reduce the number of required boreholes,
- Develop predictive models of soil behavior,
- Adapt interventions in real time based on detected conditions.
This shift from reactive to predictive geotechnical engineering not only enhances the quality of studies, but also enables more efficient management of human, material, and financial resources.
Data at the heart of the process
This paradigm shift relies on a simple yet essential foundation: data.
In the geotechnical field, data primarily comes from soil investigations. These physical tests, carried out in the field, generate mechanical measurements that feed the entire analysis chain.
But for artificial intelligence to deliver reliable results, the input data must be consistent, reproducible, and truly representative of on-site conditions. This requires strict control over the parameters measured during testing.
However, these parameters—tool advancement speed, applied pressure, rotation speed, and torque—can vary significantly depending on the operator, the equipment, or site conditions. Such variability introduces uncertainty that can reduce the usability of the data.
Standardization and semi-automatic control: towards more reliable data
To ensure consistent data quality and simplify analysis, an innovative solution has been developed: a semi-automatic drilling control system combining proportional electrohydraulic technology with machine learning.
This approach significantly increases the reliability of results. It enables more accurate interpretation of lithological transitions, improved repeatability of tests, and more consistent data collection across different sites.
Predictive analysis and historical data utilization
Another major advantage of this approach lies in the intelligent use of existing databases.
By cross-referencing data from a new project with that of previous studies—whether from internal or open sources—machine learning can:
- Identify local or regional geological trends,
- Detect recurring risk factors,
- Suggest tailored intervention scenarios.
This ability to learn from past experience and build on accumulated knowledge…
The limits of artificial intelligence: the central role of human expertise
Despite its potential, machine learning should not be seen as a standalone solution.
It is a decision-support tool, meant to enhance human practices—not replace them.
Data interpretation, contextual understanding, and critical decision-making must always remain in the hands of field professionals: engineers, technicians, and drillers. Their experience, intuition, and ability to assess the broader context cannot be replaced by any algorithm, no matter how advanced.
No matter how powerful the tool, it does not “see” the field. It does not grasp the specific constraints of a site, nor the regulatory or economic stakes involved. It is an amplifier of intelligence—but not an autonomous one.
A new era for geotechnics
The convergence of geotechnics, digitalization, and artificial intelligence is shaping a new era in ground engineering.
More than just a technological contribution, it represents a true methodological evolution.
This approach enables:
- Improved quality and reproducibility of studies,
- Optimization of drilling costs and timelines,
- Better anticipation of risks and site uncertainties,
- More strategic use of collected data,
- Skill enhancement within teams—freed from repetitive tasks and empowered in their analytical role.
This hybrid model, combining intelligent automation with human expertise, forms the foundation of modern geotechnics— durable et tournée vers l’avenir.